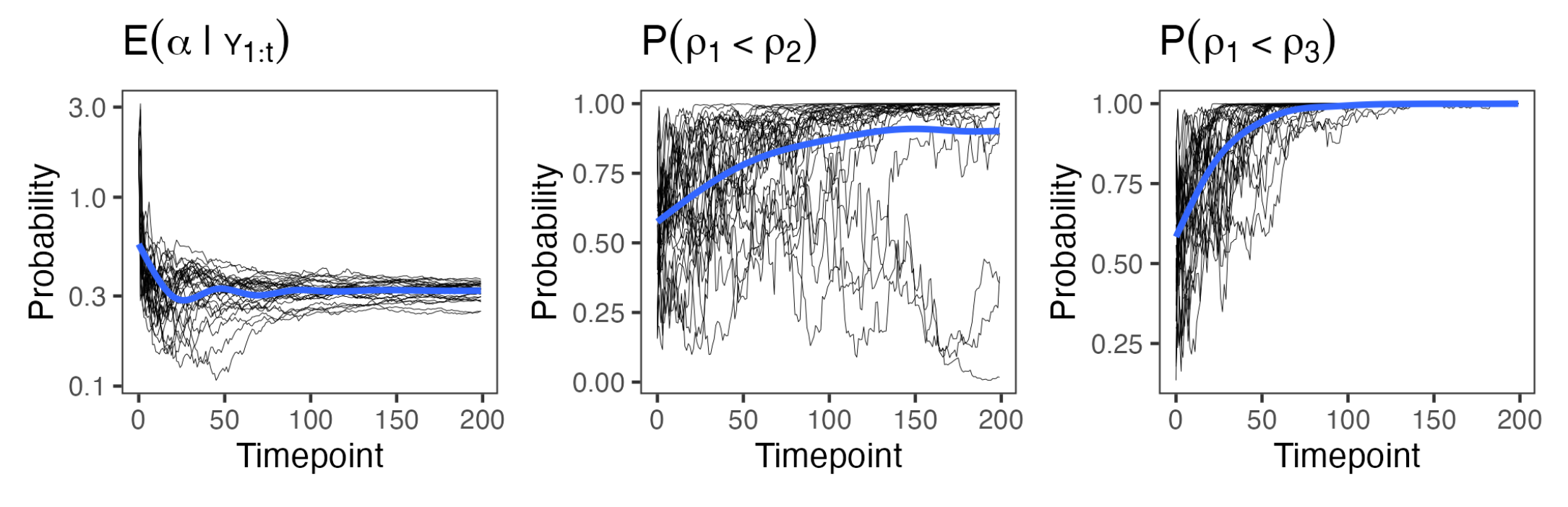
Sequential Rank and Preference Learning with the Bayesian Mallows Model
This paper considers rank and preference modeling for the case in which data arrive sequentially, rather than in a batch. The goal is to compute the posterior distribution incrementally in time, so that it can be quickly updated when new data arrives. To this end, we develop a sequential Monte Carlo algorithm for the Bayesian Mallows model. arXiv preprint currently under revision.